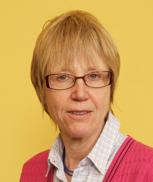
Professor
Nancy
Cartwright
Professor Nancy Cartwright
is a philosopher and methodologist of science. She currently works in the Department of Philosophy, Logic and Scientific Method at the London School of Economics, where she launched their now outstanding programme in philosophy of economics, and part-time in the department of Philosophy at the University of California at San Diego, where she works with historians and sociologists in the Science Studies Program. |
From
Science
to Policy:
What's so good about evidence?
When it comes to evidence-based policy, viewpoint matters. Typical advice tells us what counts as good science, not how to use that science to arrive at good policy.
Bike helmets certainly work – unless bikers begin to ride more dangerously and drivers perceive less risk in hitting the biker. A venture capitalist will ask for at least 20-30 separate pieces of causally relevant evidence before investing in a new business start-up.
The gold-standard of evidence-based medicine – the randomized-controlled-trial – is a bad way of using evidence to predict if policies will work in practice.
This talk shows how to do better.
Terry's Takes
(Short provocative video blogs as background to Evidence-based Policy)
|
Evidence-based Policy
The first example is about the effectiveness of laws mandating the use bicycle
helmets.
Bicycle Helmet Example. Vigorous debate regarding the efficacy of bicycle
helmets to reduce head injury has been published in the pages of the British
Medical Journal.10 Case-control studies suggest that cyclists wearing helmets
have fewer head injuries than cyclists not wearing helmets, whereas time-series
studies in jurisdictions that have passed helmet laws do not show a clear
decrease in the rate of head injuries after helmet laws have been implemented,
and in some cases these studies suggest an increase in head injuries after the
law is implemented.
At first glance this is paradoxical. Our intuitions, supported with evidence from
case-control studies, say that helmets should reduce head injuries, whereas
helmet compulsion laws fail to show much benefit and in some cases possibly
show an increase in head injuries.
There are methodological reasons that could partly explain the differences
between these studies. A worry about confounders in the case-control studies
could exaggerate the estimated efficacy of helmets: There is some evidence
suggesting that helmet wearers are overall safer bicycle riders, are involved in
less severe accidents, are richer, and more likely to be white. A worry about
confounders in the time-series studies could dampen the result of introducing
helmet laws. In some jurisdictions, helmet laws were introduced concomitantly
with safety measures, and over the periods of these studies there have been
more cars on roads, and these cars have increased in size and speed.
Leaving aside a discussion of the methodological quality of case-control
studies versus time-series analyses, this paradox can be understood by thinking
about INUS conditions. The case control studies give one piece of a causal pie:
Helmets can cause a reduction in head injuries. But those studies don’t tell
about the other pieces of the pie, that is, other factors that are causally relevant
to a cyclist’s head injury; things like driver behaviour, cyclist behaviour, and
road conditions. Now, there is evidence to suggest that at least some of these
things change with helmet wearing. Drivers give less space to cyclists who
are wearing a helmet, and cyclists take more risks (a ‘false sense of security’
phenomenon). So helmet compulsion laws don’t just change one piece of a
causal pie, they change several pieces. And that could partly explain the
differences between the two kinds of studies.
The nice thing about this bicycle example is that it illustrates two lessons at once.
First, the importance of identifying the other INUS conditions that go into a sufficient
cause, i.e., the other slices in the same pie – which one can think of as ‘helping factors’ necessary in order for the policy lever to work: Helmet wearing in combination with usual driver behaviour will decrease head injuries from bicycle accidents; helmet wearing with more dangerous driving may increase head injuries.
Second, it reminds us that in thinking about INUS conditions we need to pay attention to the unintended consequences of our actions. In implementing a policy we may not only produce unwanted side effects; we can, as in this case and in the Lucas example to be discussed, introduce factors that undermine the effectiveness of the very policy lever we employ. Of course we will always be plagued by uncertainty. We are in no position to predict many of the unintended outcomes of our policies. But some we can predict, if only we think about them in the right way.
INUS conditions: I introduce this odd technical term, INUS conditions, from philosophy because usually when we discuss policy we focus on a single cause, a single INUS condition. But we won’t be able to predict the effect of that cause without considering all the other INUS conditions and the relations among them.9 Thinking in terms of INUS conditions then serves several purposes:
· It focuses attention on the fact that there are usually a number of distinct causal
complexes that contribute independently to the effect.Sometimes we are only interested in estimating what difference the policy will make and even then
sometimes only the direction of change so that we can get by without an estimate of size. For that we clearly need somewhat less information. This is discussed in Part IV.
· It focuses attention on the other factors that are necessary along with the policy
variable if the policy is to have any effect at all.
· It focuses attention on the functional form of the relations of the variables
within a single causal complex.
· It focuses attention on the overall functional form: How do the separate causal
complexes combine? Recall my earlier remark. Often in social contexts we
assume additivity. But that doesn’t work in even simple physical cases. The
vector addition of classical mechanics is after all a long way from the simple
linear addition of effect sizes.
INUS: An INUS condition is an Insufficient but Non-redundant part of an
Unnecessary but Sufficient condition. (Non-redundant = necessary)
|
Learning Objectives
Once you complete a reasonable exploration of the clues and questions raised in this Study Guide and attended the presentation by Nancy Cartwright on THURSDAY February 10th you will be prepared:
|
Glossary
Randomized controlled trial: (RCT) A study in which people are allocated at random (by chance alone) to receive one of several clinical interventions. One of these interventions is the standard of comparison or control. The control may be a standard practice, a placebo ("sugar pill"), or no intervention at all. Someone who takes part in a randomized controlled trial (RCT) is called a participant or subject. RCTs seek to measure and compare the outcomes after the participants receive the interventions. Because the outcomes are measured, RCTs are quantitative studies.
In sum, RCTs are quantitative, comparative, controlled experiments in which investigators study two or more interventions in a series of individuals who receive them in random order. The RCT is one of the simplest and most powerful tools in clinical research.
Evidence-based policy is public policy informed by rigorously established objective evidence. It is an extension of the idea of evidence-based medicine to all areas of public policy. An important aspect of evidence-based policy is the use of scientifically rigorous studies such as randomized controlled trials to identify programs and practices capable of improving policy relevant outcomes. However, some areas of knowledge are not well serviced by quantitative research, leading to debate about the methods and instruments that are considered critical for the collection of relevant evidence. Good data, analytical skills and political support, as such, are seen as the important elements.
A causal model is an abstract model that uses cause and effect logic to describe the behaviour of a system.
Public policy can be generally defined as the course of action (or inaction) taken by the state with regard to a particular issue. Other scholars define it as a system of "courses of action, regulatory measures, laws, and funding priorities concerning a given topic promulgated by a governmental entity or its representatives." Public policy is commonly embodied "in constitutions, legislative acts, and judicial decisions."
Venture capital (VC) is financial capital provided to early-stage, high-potential, growth startup companies. The venture capital fund makes money by owning equity in the companies it invests in, which usually have a novel technology or business model in high technology industries, such as biotechnology, IT, software, etc. The typical venture capital investment occurs after the seed funding round as growth funding round (also referred as Series A round) in the interest of generating a return through an eventual realization event, such as an IPO or trade sale of the company.
In addition to angel investing and other seed funding options, venture capital is attractive for new companies with limited operating history that are too small to raise capital in the public markets and have not reached the point where they are able to secure a bank loan or complete a debt offering. In exchange for the high risk that venture capitalists assume by investing in smaller and less mature companies, venture capitalists usually get significant control over company decisions, in addition to a significant portion of the company's ownership (and consequently value).
|